AI - Risks
In addition to the many good opportunities and high potential benefits of artificial intelligence in production technology, there are also risks that need to be considered when implementing such capability tools.
On the one hand, there are risks with regard to a possible malfunction of “artificial intelligence” and the resulting loss of productivity and profitability. Malfunctions, in turn, originate from improper mathematical modelling (algorithms), incorrect parameterization (production coefficients) of these algorithms and an insufficient amount of data (good data) in line with requirements to ensure that the mathematical mapping is always self-optimizing and correct, see also the previous chapter.
Users of digitalization technology are definitely faced with the following questions during the application of an artificially intelligently controlled production environment, which should at best be addressed preventively during the planning phase of such projects:
- Was the optimization goal chosen correctly and is it still valid?
- How well is the optimization goal achieved (goal evaluation)?
- Who evaluates the optimization quality?
- Which boundary conditions in the production system relevant to the optimization target have changed?
- How accurate was the plausibility check of the mathematical model (validation)?
- When was the last valid validation of the mathematical model carried out?
- Is the plausibility checker's statement comparable?
- Is there clear documentation of the plausibility check / validation (audit)?
- Have the right algorithms been selected for machine learning?
- Are these algorithms (type & kind) still suitable for the optimization goal?
- Are the algorithms checked, monitored, their optimization quality and capability tested and improved at regular intervals?
- Are the control variables (production coefficients) correctly selected or do they have to be readjusted to the user's changed production conditions?
- Are there any programming errors within the mathematical model?
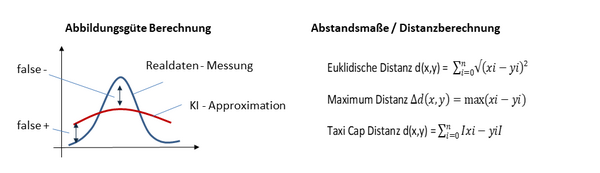
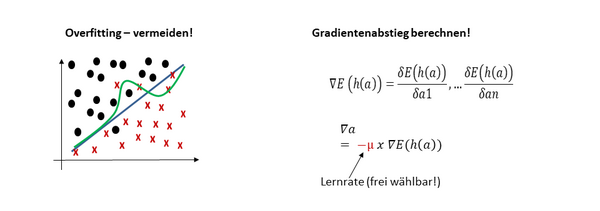
- Where do I obtain the AI hardware, the data acquisition system?
- Who guarantees the sustainable function of the data acquisition system and its software updates?
- How do you want to guarantee the availability of the data acquisition system - in-house or external service?
- Who evaluates the development of information quality?
- Is it an in-house solution or the solution of a service provider?
- Who adapts the hardware - how high is the potential for possible sources of error due to outdated or worn hardware?
- Who evaluates these digitalization issues in your company - How confident are employees in their statements - How can it be monitored?
- Is there a sufficient level of expertise in setting up the digitalization infrastructure?
- Has this expertise been tested?
- Are methods of deductive or purely inductive learning already being used?
- What is the deductive approach based on - are there any sources of error with regard to the expert knowledge used?
- Is the technical theory as the knowledge base of the deductive learning approach of your artificial intelligence fully valid? Do changed production boundary conditions have to be checked regularly and their influence as a cause of error excluded?
- Can the issue be controlled in the long term? Or does the production control system become unintentionally independent?
- Do the sensor solutions used function sustainably and with what error rate? -> How does the quality of information develop over time?
If such questions remain unanswered, they provide an initial framework for assessing the risks of using such optimization assistance. It is important to find a healthy compromise with regard to the ability of in-house performance in the context and at the same time the acceptance of external service providers (e.g. the hardware of data acquisition systems) without becoming dependent on individual employees or external third parties and without losing production information (your production know-how) to third parties.
Products relevant to this article:
GRX-Q
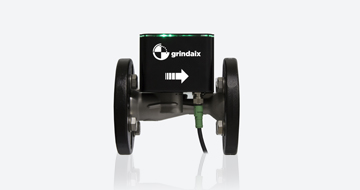
You may also be interested in these articles from our magazine:
AI - What is it?
Basically, the term artificial intelligence is a technical misnomer in this context. Artificial intelligence describes the academic endeavors of humans to research the human brain and its performance and to reproduce it with the help of computer-aided methods.
AI - obstacles
The biggest obstacle to the implementation of digitalization technology at present is the often prevailing confusion among decision-makers responsible for production as to how the project should even be approached.
AI - challenges
As already described in Chapter 3 (AI - How to proceed), the algorithms for the successful application of machine learning in production technology require as much good data as possible. Good data is data whose values are generally as correct as possible.
Frequent problems during grinding
Modern high-volume production facilities for metal components often have large and equally complex cooling lubricant systems. Keeping an eye on all parameters and ensuring reliable operation is therefore no trivial matter. Coolant monitoring systems can be used to support the monitoring of the cooling lubricant system.