AI - obstacles
The biggest obstacle to the implementation of digitalization technology at present is the often prevailing confusion among decision-makers responsible for production as to how the project should even be approached and how much time and money it will cost and what measurable benefits it will bring. The question arises as to whether such a production optimization system can be amortized in general and over time. Fears are stirred up and hopes are spread in numerous media channels without, however, being able to make concrete promises of benefits. How can we keep a clear head?
In this orientation article, we want to list and, if possible, evaluate the main fears and possible obstacles.
The basic opinion of most production companies, also due to their obligations under company-related confidentiality and data protection regulations, is that all production data and information must not be passed on to third parties or to external data systems (cloud, external IT services, Internet) in order to protect against loss of know-how.
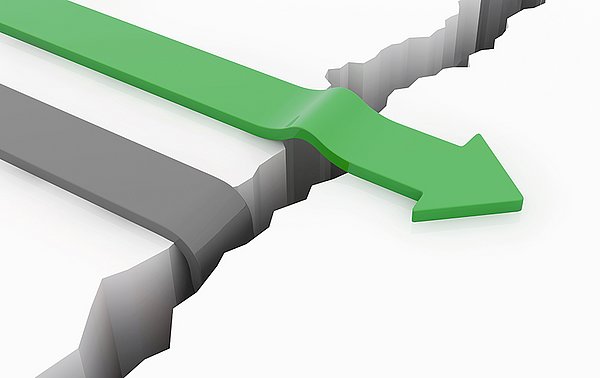
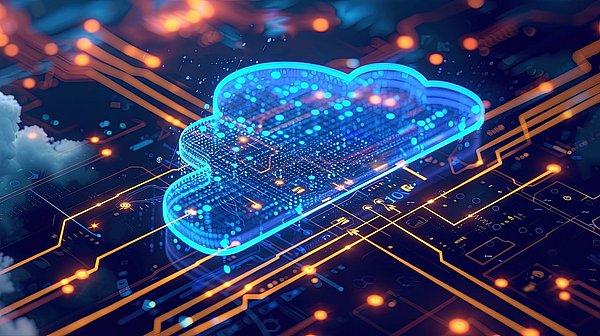
In addition, it must be ensured at all times that installed operating systems are protected as far as possible against unauthorized access from “outside” (“hacking”). For example, WLAN-enabled sensors and devices may only work with encrypted data so that it cannot be accessed and used without authorization. On the user side, there is usually a desire for a practical and robust data acquisition system that is also as secure as possible as an in-house solution. WLAN-capable sensors and devices are often inadequate for use in production systems due to the high strength of electromagnetic fields that exist there. In addition, WLAN-capable sensors often have to be equipped with a power supply, so that networking via this power line would already be possible without transporting signals via a relatively insecure WLAN network.
Cloud-based solutions promise the optimal use of artificial intelligence and secure data storage. However, decision-makers responsible for production are reluctant to move their production experience to the internet because it is perceived as an insecure place. The in-house solution appears to be the most secure solution at the moment.
Lack of skills - a major obstacle
Another major obstacle is the lack of availability of specialist staff. Small and medium-sized companies in particular cannot rely on central IT departments to deal with the rapid implementation of digitalization. There is a lack of time and technical expertise to address the issue internally. However, the use of consulting firms does not appear to be the most effective solution in this context. What is needed are companies with a hands-on approach that offer fast and reliable implementation while keeping the financial risk of the production company as low as possible. This can be ensured by allowing the production company to test and experience a data acquisition system free of charge before deciding to purchase one. A period of use of up to 6 months is usually recommended in order to build up a reliable basis of experience.
However, it is not only the hardware that is needed, but also the industry-specific expertise to define and monitor limit values, keep error logs and make them efficiently usable, instead of despairing at an excessive number of error messages.
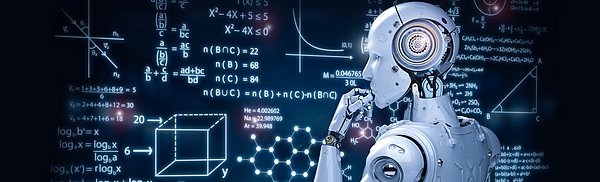
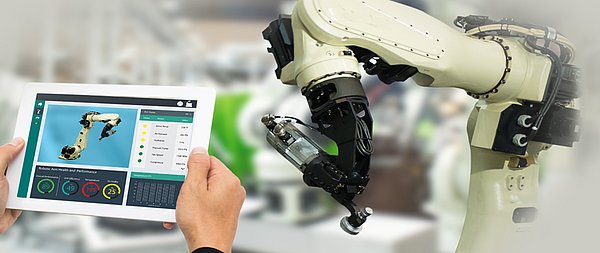
Legal issues pose further obstacles. Who is liable for production losses if algorithms do not work efficiently and effectively and the optimization target is not achieved in the desired or agreed time? The legal basis is not yet sufficiently prepared for these developments due to the lack of experience. Can sales promises that are made as part of a digitalization project for autonomous production even be fulfilled? Unfortunately, the answers to these questions must first be experienced first-hand. There is almost no supplier of a digitalization solution that can define a concrete value proposition as an assessable performance feature. Such a vagueness of project success statements and thus concrete project risks cannot be clearly eliminated in the run-up to a digitization project and therefore remain over the course of the project as long as no positive experiences are made.
Willingness to take risks - the solution to the problem?
This requires a certain degree of risk-taking on the part of the decision-makers responsible for production. As decisions are only made in groups and rarely by one person, there are delays in implementation. The market virtually blocks itself. There are no insurance policies or guarantees. Does this mean that only the courageous, risk-taking type of entrepreneur who takes responsibility and invests money in an uncertain outcome can be considered a “first mover”? Not quite, at least up to the performance level of limit value monitoring for properly functioning predictive maintenance (see chapter 2), reliable promises can be made about the added value of the system. Performance promises only become vague from the moment that software-based mathematical modeling is used as a decision-making aid.
This means that the recommendation already made in Chapter 1 remains valid.
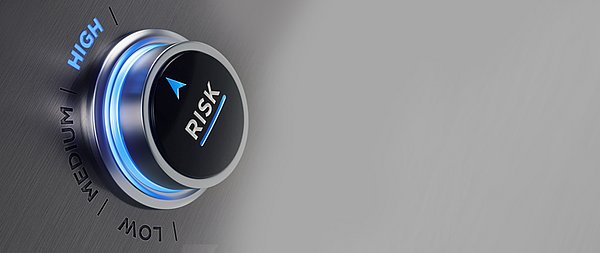
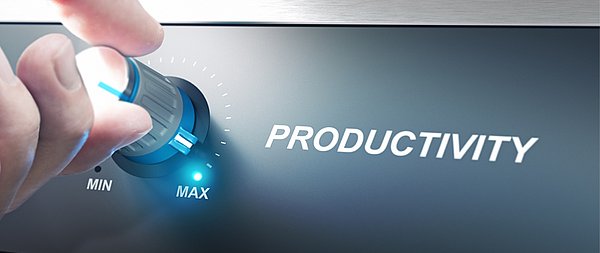
Data should first be recorded, analyzed, observed and understood in real time before it can be evaluated or autonomously controlled using ML! Potential benefits and added value arise from handling and experience in the application. They can almost never be predicted completely or with absolute certainty. The journey becomes the destination - just like in real life.
As a positive example, however, it should be mentioned here that the possible installation of such a digitized production monitoring system can significantly reduce the reject rate (e.g. reduction of thermally damaged components) and production downtimes through a predictive maintenance approach that precedes the use of artificial intelligence, so that amortization of the investment costs can be achieved after just one single damage event (reject - blocked production quantity) avoided by means of this digital “simple” assistance. The scrap costs are usually significantly higher than the total investment in a suitable production monitoring system with a possible AI connection.
This would significantly reduce the risk of whether the use of artificial intelligence defined as a long-term goal within production control generates measurable benefits, as amortization can already be achieved in the early project phase of predictive maintenance. All possible production advantages that arise in a later project phase would therefore be seen as an additional increase in profitability or improvement of the selected, company-specific optimization parameter. The “predictive maintenance approach”, which can now be implemented with certainty by using the data acquisition system described above, would thus serve as the economic justification for starting a project to generate artificial intelligence in production systems.
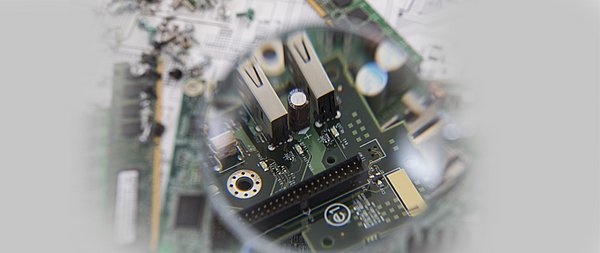
Products relevant to this article:
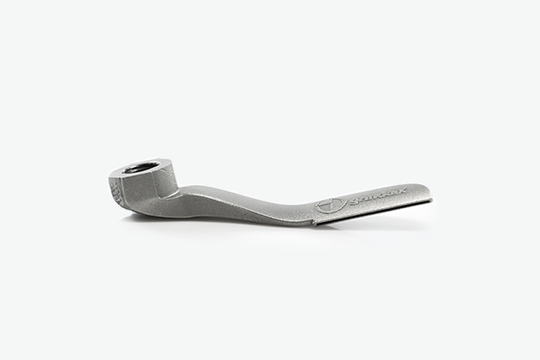
You may also be interested in these articles from our magazine:
AI - challenges
As already described in Chapter 3 (AI - How to proceed), the algorithms for the successful application of machine learning in production technology require as much good data as possible. Good data is data whose values are generally as correct as possible.
AI - Implementation
The following example demonstrates how a digitalization project can be successfully implemented.
AI - Risks
In addition to the many good opportunities and high potential benefits of artificial intelligence in production technology, there are also risks that need to be considered when implementing such capability tools.