AI- Autonomous production
Production technology is increasingly characterized by the use of computer-aided knowledge management systems. A distinction is made between expert systems, which pursue a deductive learning approach based on empirical knowledge, and inductive optimization methods, which are based on mathematical models (algorithms), initially without expert knowledge, and function in a self-learning manner towards a clearly defined optimization goal. The use of inductive learning methods (deep learning, machine learning) has the potential to become the key technology for autonomous production in the future. Autonomy should be understood here to mean that the future production unit (machine network including automation technology across the entire production chain) is controlled and regulated fully automatically by a computer in a highly efficient and effective manner without human intervention in terms of productivity, quality and cost-effectiveness.
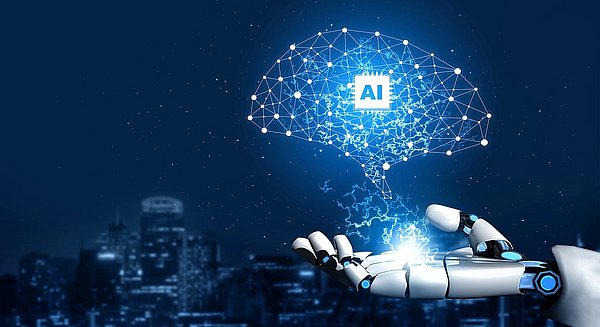
The vision of autonomous production
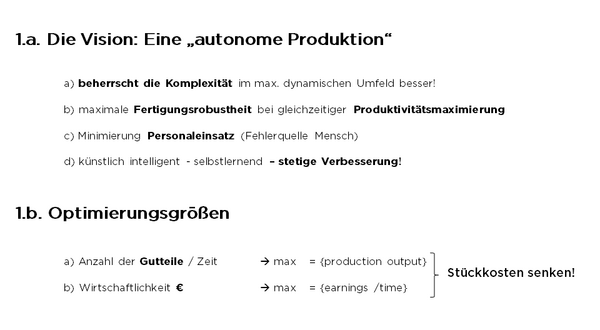
Self-learning electronic data processing systems master the increasing complexity in the environment of dynamically operating manufacturing systems much better than individual humans and even entire groups of humans can, thanks to the currently widely available computing power of the associated hardware. In contrast to individual humans, they work constantly, increasingly error-free, reliably and, with the help of predictive assistance systems, achieve maximum production robustness while maximizing productivity at the same time. This will not necessarily reduce the number of people required in production, but will enable humans to support the system as much as possible thanks to the wide range of options available to them. In this type of system, humans will be able to act either as workers without authority or, as proven experts, as plausibility checkers of the decisions made numerically.
Computer-controlled production requires concrete optimization targets, otherwise the classic learning methods of artificial intelligence or machine learning cannot be applied. Such targets can concern the number of good parts per hour or economic efficiency.
Priorities must be assigned. For example, the primary objective may be to reduce unit costs. Without the simultaneous target value specification of the number of pieces to be produced per time unit, the system may not work effectively.
One specific optimization goal is, of course, to maximize the number of good parts per hour in order to make production more effective and efficient. In this way, unit costs can be reduced.
Maximizing the number of good parts / hour = max {production output}
Profitability as a specific optimization target also contributes greatly to better production and lower unit costs.
Maximization of economic efficiency (€) = max {earnings per hour}
Products relevant to this article:
GRX-Q
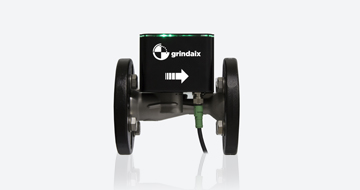
You may also be interested in these articles from our magazine:
AI - What is it?
Basically, the term artificial intelligence is a technical misnomer in this context. Artificial intelligence describes the academic endeavors of humans to research the human brain and its performance and to reproduce it with the help of computer-aided methods.
Cooling lubricant pressure control systems
Pressure control systems are particularly relevant for large and complex cooling lubricant supply systems. We have compiled the advantages over conventional pressure control systems and outline the technical implementation of an adequate pressure control system.
Frequent problems during grinding
Modern high-volume production facilities for metal components often have large and equally complex cooling lubricant systems. Keeping an eye on all parameters and ensuring reliable operation is therefore no trivial matter. Coolant monitoring systems can be used to support the monitoring of the cooling lubricant system.
AI - How do you proceed?
The first step in this context is to start implementation as soon as possible and not to wait until a competitor can present a solution. Because this solution, if it is available at all, certainly cannot simply be copied or purchased at the touch of a button.